AI/ML B2B ENTERPRISE MANUFACTURING
Empowering Users with AI: Simplifying Root Cause Analysis and Issue Containment.
I helped shape and improve the experience of an AI-driven tool for the engineers to reduce workload and increase productivity. This AI/ML predictive analytics tool was developed internally to support manufacturing plants.
* Due to NDA, some details have been simplified or omitted.
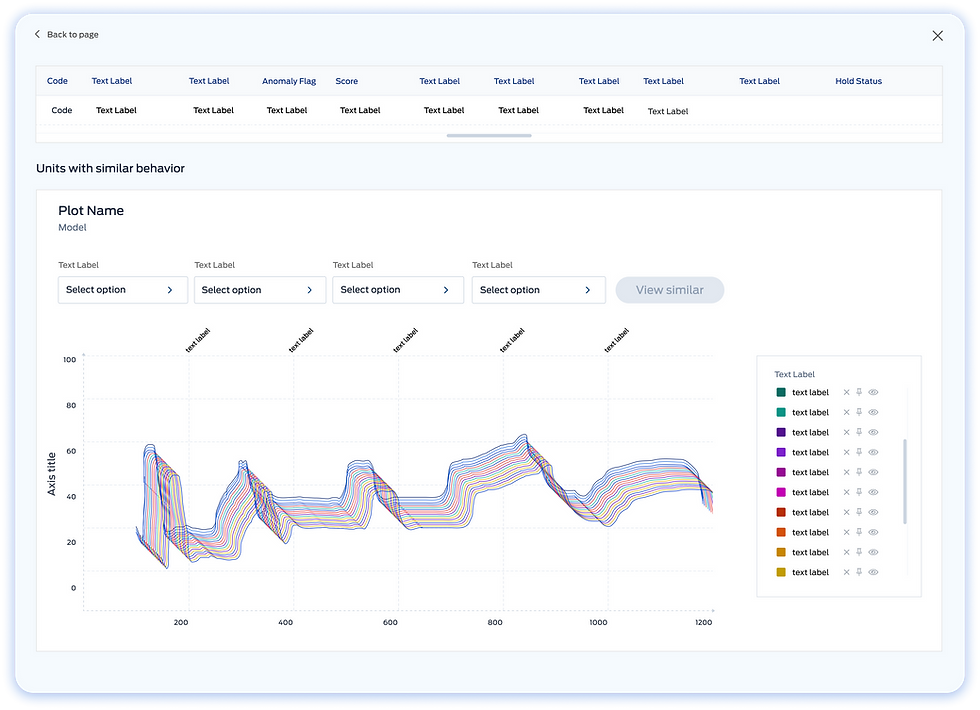.png)
OVERVIEW
In manufacturing, where quality issues can cost millions, early problem detection is vital. While AI/ML tools are revolutionizing quality control, they must support—not burden users.
Existing AI/ML tool overwhelmed users, hindering adoption. To fix this, my team developed a user-centric AI solution, with my role focused on user discovery and end-to-end design, balancing cross-functional needs.
The new approach streamlined anomaly investigations, reducing manual work and allowing engineers to focus on critical problem-solving.
TEAM
Data scientists, PO, PM, tech anchor, software engineers, data engineers
MY ROLE
Lead Product Designer
TIMELINE
2024
OUTCOMES
30% Faster Investigations
Increased Trust in AI
Higher Adoption Rates
The Problem
In manufacturing, catching quality issues early is crucial to reducing warranty costs.
The existing AI/ML app, designed to assist plant engineers in identifying and addressing these issues, often added to their workload instead of reducing it. This led to inefficiencies and low adoption among users who found the tool burdensome and unintuitive.
Context
Engineers faced challenges across two key use cases:
1) root cause analysis 2) issue containment
%20(1).png)
Use Case 1 - Root Cause Analysis (Engineer A)
I need to determine which AI flagged anomalies are more likely to become a real problem, so I can prevent it.
To determine that, the user has to pick an anomaly (based on a specific characteristic), review it, compare it against other anomalies.
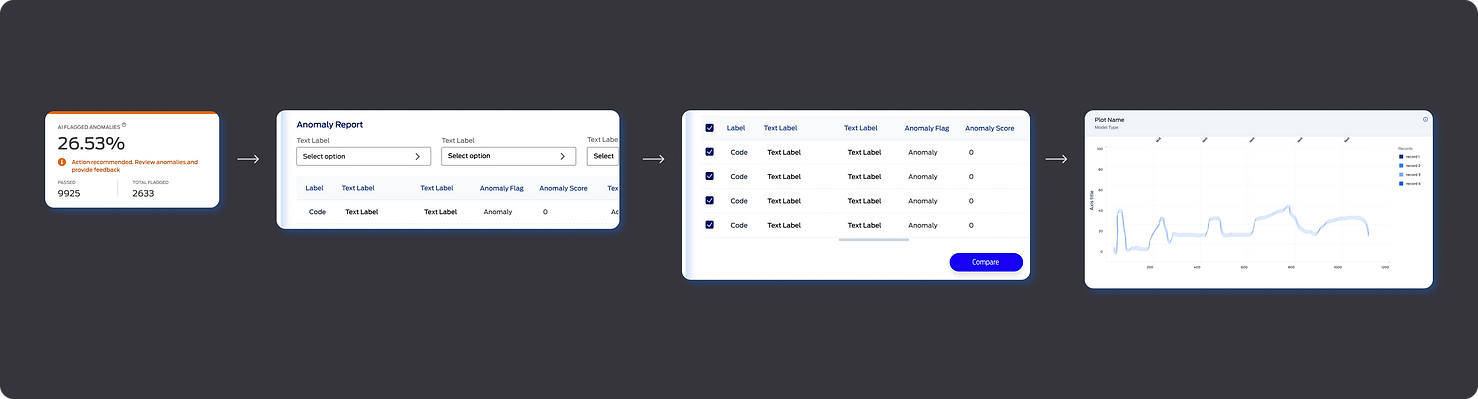%20(1).png)
Old experience
AI TECHNOLOGY PROBLEM
The problem with some AI systems, especially in anomaly detection, is that it may identify where an anomaly occurs but not why it happens, requiring users to spend additional time investigating and troubleshooting.
The human has to investigate to confirm whether that anomaly is an actual real problem or whether AI is inaccurate in its prediction (false positive). This problem led to:
-
Repetitive manual comparisons to investigate anomalies.
-
Isolated investigations with limited pattern recognition.
-
AI limitations requiring anomalies to share specific characteristics for comparison.
-
Reliance on the temporary POC app, which was only a stopgap solution.
AI technology limitations caused usability challenges, including restricted functionality in the app interface.
Time-consuming workflows reduced user confidence and trust in the AI system which is critical for the adoption of the AI tools - the goal we were trying to achieve to get a buy-in from the plant.
Use Case 2 - Issue Containment (Engineer B)
I need to find AI-flagged anomalies similar to confirmed real problem, so I can prevent issue spread.
To find it, the user has to view all anomalies that meet the same one characteristic (that define similarity) as the confirmed problem .
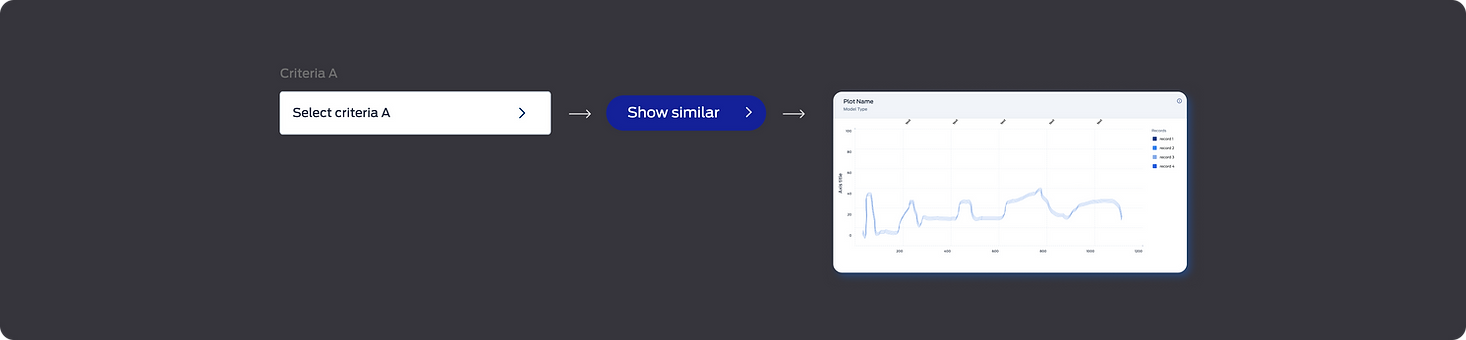%20(1).png)
Old experience
This way of viewing anomalies was:
-
time consuming to review potentially hundreds of anomalies (another AI technology problem with over flagging)
-
limited to only one characteristic for comparison
-
one characteristic may not be enough to accurately determine similarity
Our AI anomaly detection tool led to missed opportunities to contain issues early.
Design Strategy
DESIGN SOLUTION
The design solution needed to simplify interfaces and workflows to reduce cognitive load and make AI outputs actionable:
-
Simplify Root Cause Analysis: Use AI to help users identify patterns without repetitive manual comparisons.
-
Enhance Issue Containment: Expand filtering options for exploring the broader impact of confirmed issues.
COLLABORATION
I worked closely with developers, product owners, and data scientists to ensure alignment with technical constraints and business objectives.
SUCCESS METRICS
-
Reduced time spent on anomaly investigations.
-
Increased user adoption of the AI tool (and not the temporary POC app)
-
Adoption by other plants
Solution - Use Case 1 (Root Cause Analysis - Engineer A)
I need to determine which AI flagged anomalies are more likely to become a real problem, so I can prevent it.
We worked to streamline root cause analysis by:
1) expanding the range of how many anomalies the picked anomaly can be compared to
2) showing anomalies with similar traits, reducing the need to manually compare individual anomalies one by one.
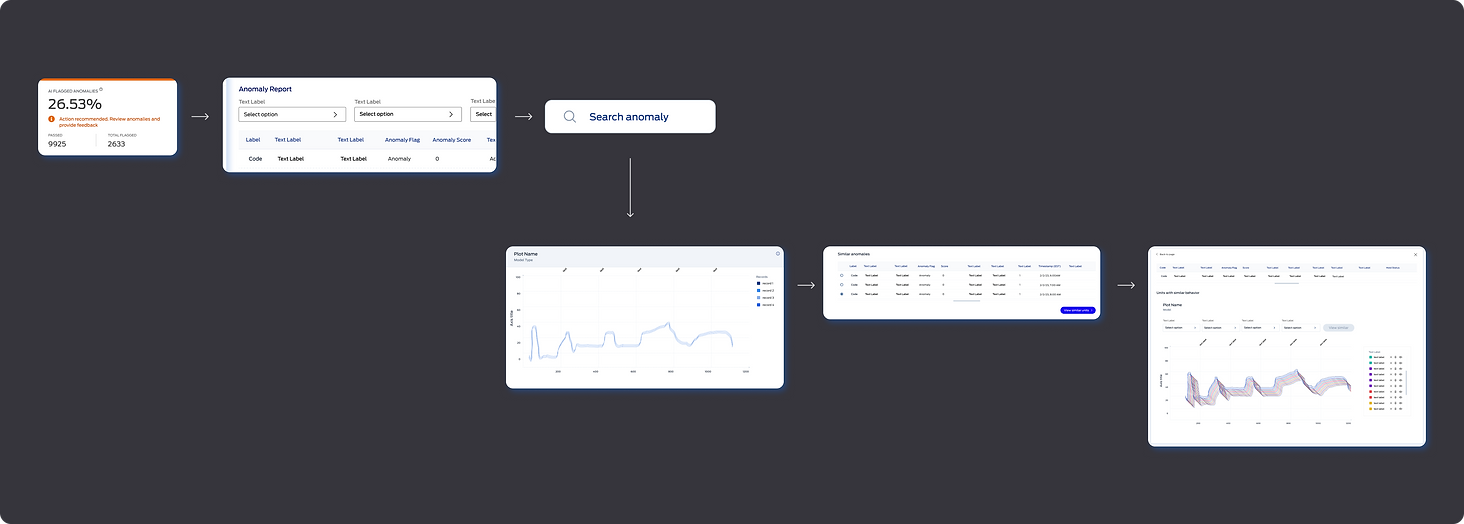%20(1).png)
New experience
Solution - Use Case 2 (Issue Containment - Engineer B)
I need to find AI-flagged anomalies similar to confirmed real problem, so I can prevent issue spread.
The users now would be able to:
-
search for a specific anomaly and view similar anomalies
-
change comparison criteria by more than one characteristic
-
compare a larger range of anomalies
-
get a better picture of whether a particular anomaly has a higher chance of being a real problem.
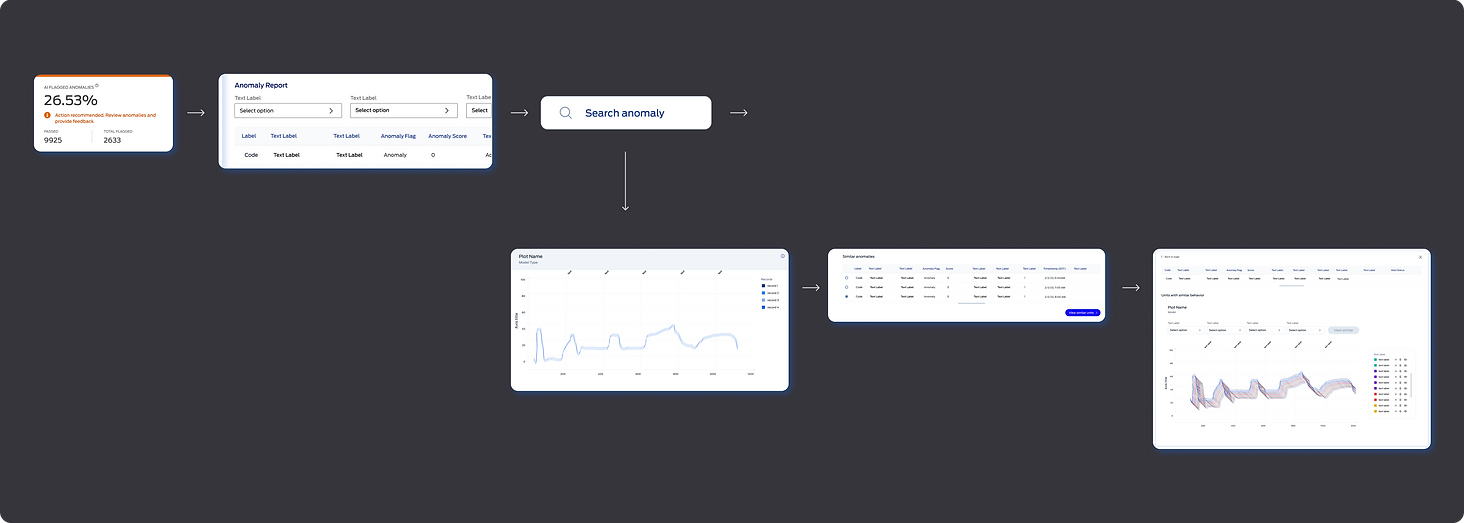%20(1).png)
New experience
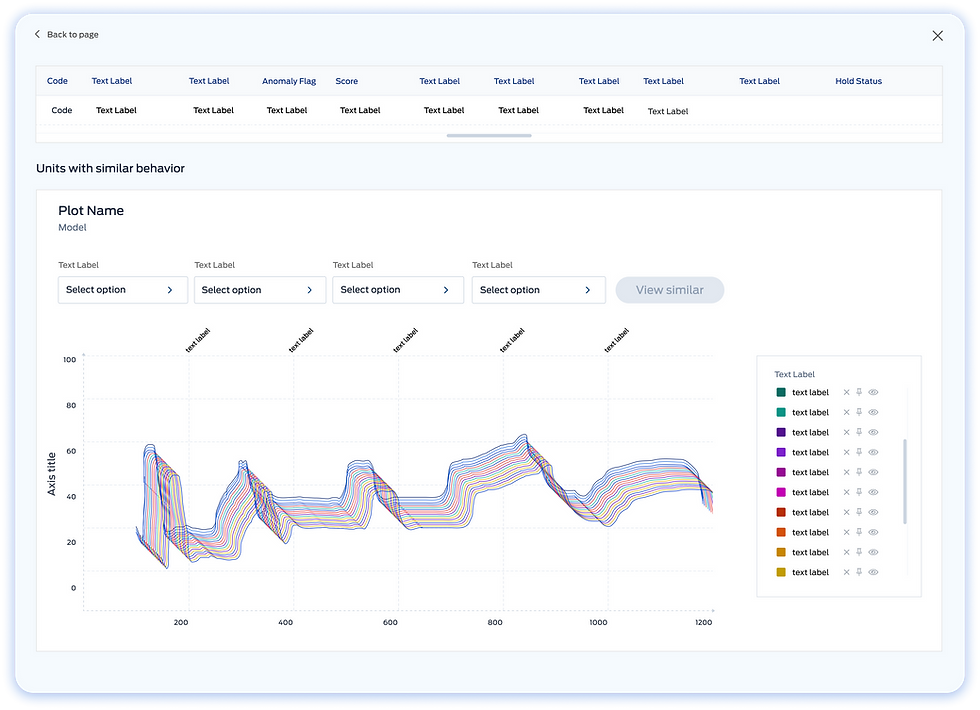.png)
Impact
-
30% Faster Investigations: Engineers saved significant time with clustering tools and expanded filtering options.
-
Less Manual Work: Repetitive tasks were reduced, allowing users to focus on high-priority issues.
-
Increased Trust in AI: Clear patterns and transparent clustering increased user confidence.
-
Higher Adoption Rates: Engineers embraced the tool in place of a temporary solution. Other plants began working with our team to implement similar solution.